Advantages:
- Dramatically reduces the impact of unlearnable noise using smart data transformations
- Recovers facial recognition accuracy from nearly 0% to over 85%
- Designed for large facial datasets with limited number of images per person
Summary:
Some facial datasets are made "unlearnable" by adding small, hidden changes that stop AI models from learning. These changes are meant to protect people's faces from being used without permission for AI model training. While this works for image classification, it doesn't work as well for facial recognition, where there are many identities and few images for each person. These tricks can cause the system to fail completely, creating a major problem for both accuracy and privacy.
This technology uses grayscale, blur, and color adjustments during training to cancel out the unlearnable noise. Tested on the popular CelebA dataset, the new training method increased recognition accuracy from 0.01% to 85.89%, even better than models trained on clean data. This shows that unlearnable data isn’t enough to protect privacy and better defense methods are needed. This solution is ideal for companies building reliable and privacy-aware facial recognition systems.
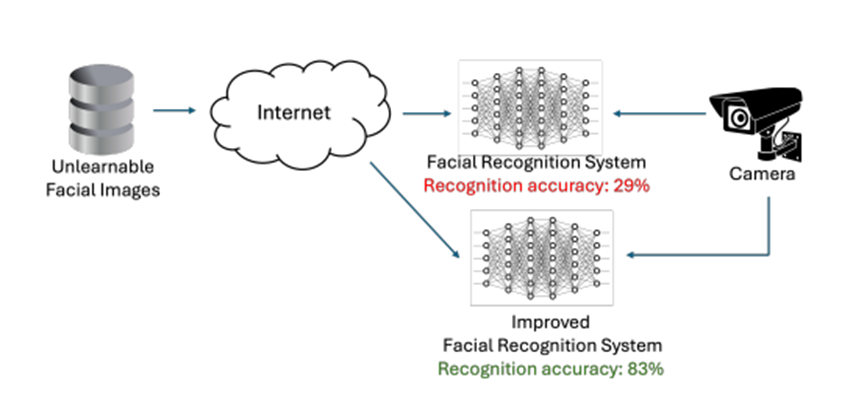
This image shows how unlearnable facial data reduces recognition accuracy and how the proposed training method restores it. The improved system boosts performance from 29% to 83%, making facial recognition reliable again even with protected or altered datasets.
Desired Partnerships:
- License
- Sponsored Research
- Co-Development