Advantages:
- Enables rapid and accurate identification of mosquito gonotrophic stages, enhancing disease surveillance efforts effectively.
- Reduces the need for extensive entomological expertise, making mosquito monitoring more accessible and efficient.
- Facilitates integration with citizen-science platforms, empowering community involvement in tracking mosquito populations and stages.
Summary:
Mosquito-borne diseases continue to pose a significant global health challenge, with increasing incidences even in regions previously considered malaria-free, such as Florida and Texas in 2023. Traditional methods of monitoring mosquito populations and their gonotrophic cycle stages require extensive entomological expertise and manual effort, making them time-consuming and less accessible. Understanding these stages is crucial for effective mosquito surveillance and control, which are essential in preventing the spread of diseases like malaria, dengue, and Zika.
Our innovative approach leverages computer vision techniques to automate the classification of gonotrophic stages in female mosquitoes, addressing this critical need. Using an EfficientNet-B0 model trained on a comprehensive dataset of 1,959 images from 139 mosquitoes across three medically significant species, our system achieved an impressive 93.59% accuracy. This breakthrough not only enhances the speed and accuracy of mosquito surveillance but also democratizes access to sophisticated analysis through potential integration with citizen-science platforms and open-access web portals like mosquitoID.org. This novel application of AI in entomology represents a significant advancement in the fight against mosquito-borne diseases, offering a scalable and efficient tool for global health efforts.
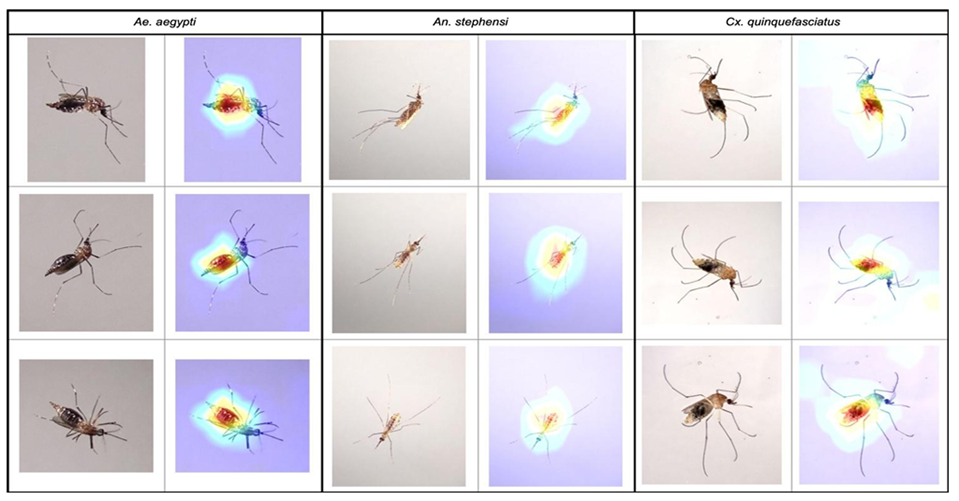
Fully fed gonotrophic stage images with Grad-CAM per species, showcasing the AI model's focus on mosquito abdomens for classification.
Desired Partnerships:
- License
- Sponsored Research