Advantages:
- Utilizes wearable devices, enriching pain datasets with comprehensive body and muscle data.
- Promotes responsible user interaction, enhancing model relevance to individual experiences in pain assessment.
- Integrates human-machine cooperation for superior predictive capabilities in pain assessment models' development.
Summary:
In the landscape of pain assessment methods, the predominant challenge stems from the limited generalization of automated techniques due to a dearth of extensive and diverse annotated datasets. Current methods also lack responsible user interaction and often disregard internal and external factors in model development. Our research introduces a novel cooperative learning framework, adept at mitigating data scarcity concerns while prioritizing user engagement and accommodating individual variations in the development of pain assessment models.
Our approach represents a notable advancement in pain assessment technology by harnessing body and muscle movement data from wearable devices. This innovative cooperative learning framework synergizes human and machine capabilities, demonstrating enhanced predictive efficacy. What distinguishes our technology is its unique ability to harmonize the experiential aspects of pain with computational precision. By fostering collaboration between human insight and machine learning, our framework not only addresses data limitations but also enables a more nuanced and personalized comprehension of pain, marking a significant stride in the evolution of pain assessment models.
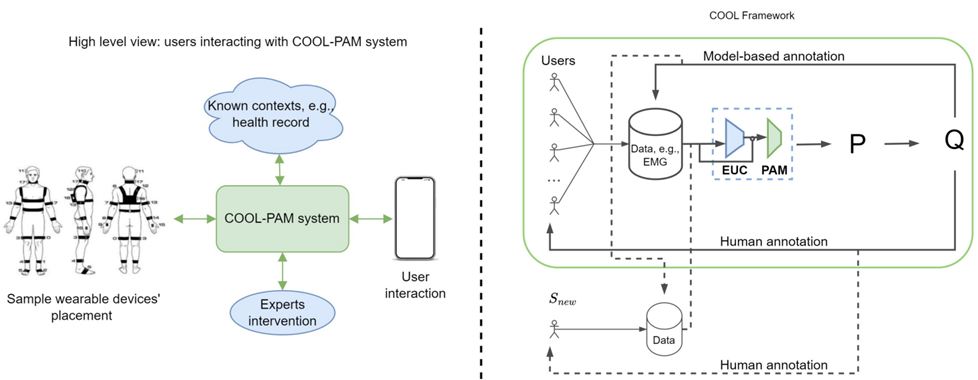
Overview of COOL-PAM system and COOL Framework
Desired Partnerships:
- License
- Sponsored Research
- Co-Development