Advantages:
- EW-Tune framework ensures robust privacy, preventing unauthorized access to sensitive AI data.
- Differing from conventional approaches, EW-Tune is custom-tailored for enhancing already established AI models, streamlining the process.
Summary:
This technology introduces EW-Tune, a pioneering solution aimed at refining AI language models. Focused on privacy, EW-Tune prevents unauthorized access to sensitive data stored within these models. Unlike conventional methods tailored for new AI models, EW-Tune is designed to optimize privacy for fine-tuning large language models (LLMs). By merging Edgeworth accountant with privacy differential privacy guarantees, EW-Tune substantially minimizes the risk of extracting private information. This leads to preventing adversaries from accessing users’ personal data. Furthermore, the methodology is openly shared, encouraging fellow researchers to explore its implementation. This collaborative approach is poised to enhance AI safety and performance for a broader audience.
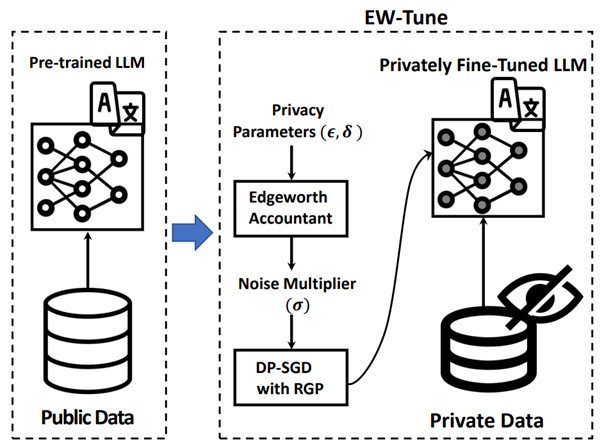
Abstract View of the Proposed EW-Tune Framework
Desired Partnerships:
- License
- Sponsored Research
- Co-Development