Advantages:
- Enhanced Accuracy: Achieves superior performance in human activity recognition by effectively harnessing spectral properties and temporal features.
- Automated Feature Learning: Utilizes deep neural networks for automatic feature extraction, saving time and resources while improving model efficiency.
- Versatility and Adaptability: Incorporates advanced data augmentation techniques, making it adaptable to diverse datasets and real-world scenarios.
Summary:
Deep Feature Learning for Human Activity Recognition is an advanced technology revolutionizing predictive analytics. Seamlessly integrating deep neural networks and unsupervised learning, this innovative solution autonomously extracts intricate features from raw biological time-series data, achieving an impressive 29.8% reduction in the overall average error-rate, surpassing conventional methods. The recent upgrade with the Sub-Transfer Learning (STLM) technique enhances adaptability for outlier users, fine-tuning parameters and significantly improving classification performance. Advanced data augmentation techniques, including SMOTE, further fortify predictive models, making them robust and efficient while eliminating the need for manual feature engineering. Streamlining model development saves valuable time and resources, making this technology applicable in diverse industries, from healthcare and sports to fitness and surveillance.
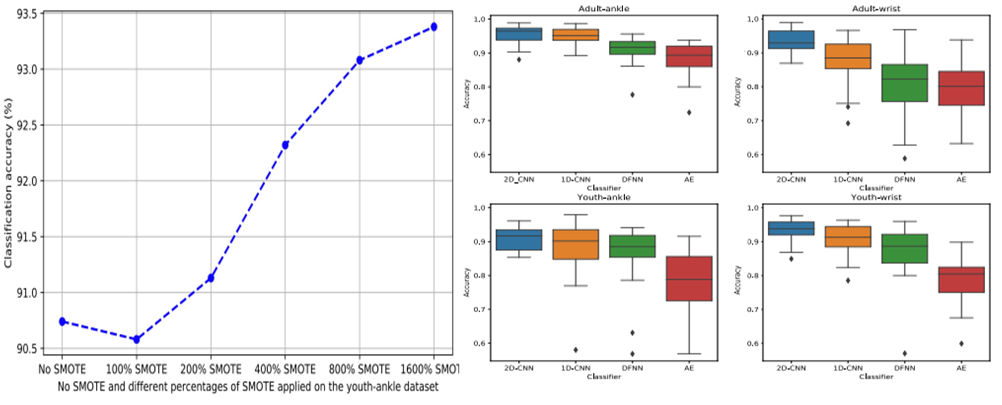
The figure illustrates the comparison between a 2D Convolutional Neural Network (2D-CNN) using spectrotemporal inputs and a method based on human-engineered features. The 2D-CNN outperforms the human-engineered features method with a significant 29.8% reduction in the overall average error-rate. Additionally, synthetic data augmentation using SMOTE is explored to further improve the performance of automated feature-learners.
Desired Partnerships:
- License
- Sponsored Research
- Co-Development