Advantages:
- Enhanced vulnerability identification
- Efficient verification process
- Proactive vulnerability detection
Business Summary:
Businesses seek innovative solutions for growth, efficiency, and digital adaptation amid the pandemic, requiring professional, trustworthy services, open communication, and continuous learning. The difficulties in locating and validating vulnerabilities in system-on-chip (SoC) architectures, which are widely employed in a variety of applications including the Internet of Things (IoT), mobile devices, and cloud computing, are addressed by this invention. The new method uses machine learning models to automatically record communication patterns between various IPs inside the SoC. The method builds these models using simulation, emulation, or post-silicon execution, and then uses adversarial machine learning techniques to produce new system communication behaviors. This method seeks to identify potential vulnerabilities that might be caused by erroneous IP addresses, mistakes, or incorrect setups. Before deploying SoC designs, the innovation provides a novel verification technique to confirm the absence of such vulnerabilities, improving system security overall.
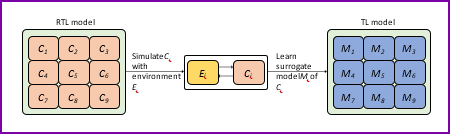
An overview of surrogate model learning
Desired Partnerships:
- License
- Sponsored Research
- Co-Development