Advantages:
- Enhanced safety through accurate AV identification.
- Improved traffic management and efficiency.
- Data-driven insights for research and business opportunities.
Business Summary:
Automated vehicles with adaptive cruise control have been operating on public roads with a significant rapidly growing market penetration. These Learning-based models achieved high accuracy, correctly identifying 98.14% of AVs and 94.19% of human-driven vehicles (HVs). The results validate the feasibility of using existing infrastructure and economic technologies for AV identification, opening data-driven opportunities for managing mixed traffic. AVs exhibit distinct car-following behaviors, as confirmed by the physics-based approach identifying over 80% of AVs and 70% of HVs. Accurate AV identification is crucial for traffic management and safety. Learning-based models are recommended for real-world applications, offering higher accuracy. Future research can address challenges like probability shifts and consider lateral movements and manufacturer-specific assessments. Overall, this study demonstrates successful AV identification and highlights the potential of existing technologies in regulating AV presence on public roads. Model improves traffic management, safety, and insurance premiums for autonomous and human-driven vehicles.
(a)
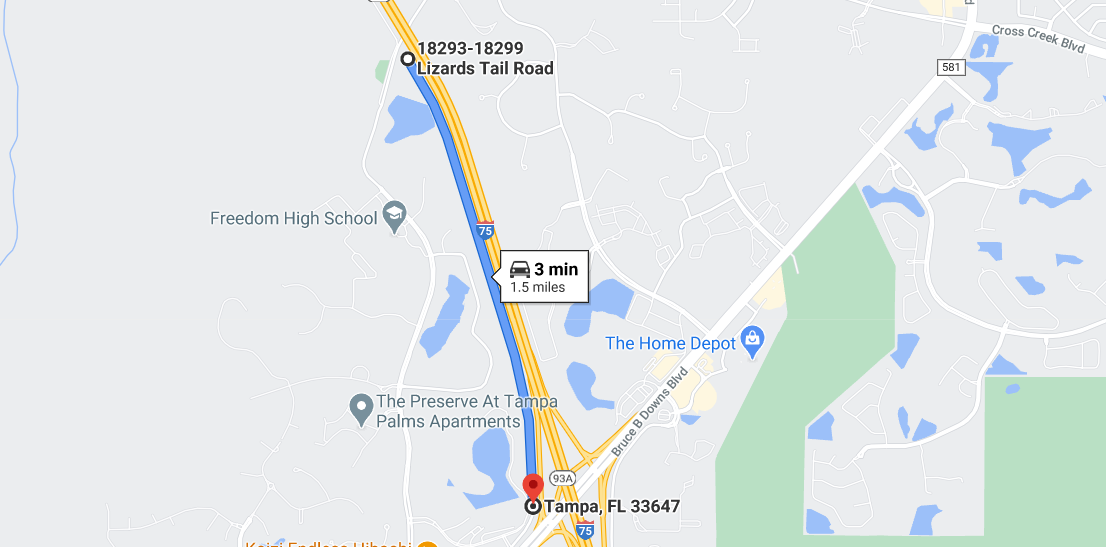
(b)
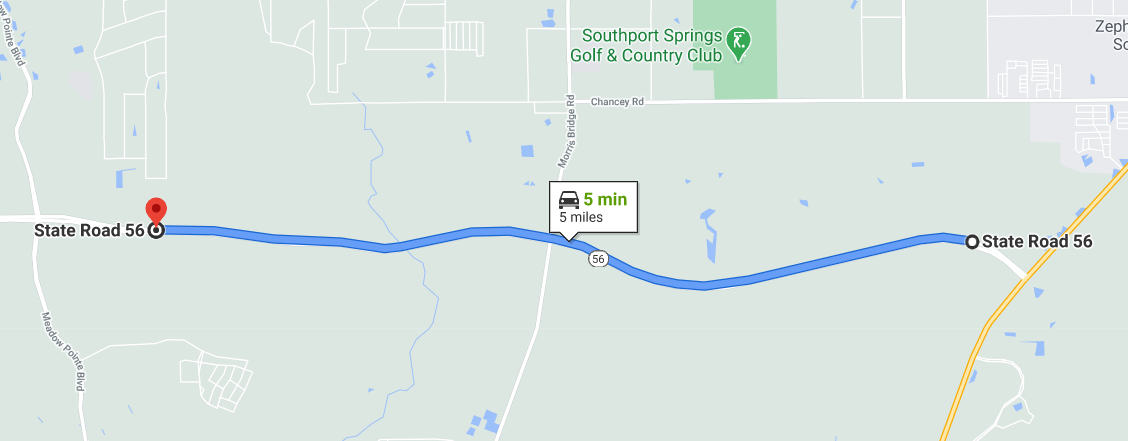
Mixed traffic experiment locations. (a) Low-speed experiment. (b) High-speed experiment
Desired Partnerships:
- License
- Sponsored Research
- Co-Development