Competitive Advantages
- Organization-specific context-based threat detection and mitigation.
- Continuous refinement of defender’s policies according to the threats identified.
- Assignment of security personnel according to the threat level of vulnerability and skills of the individual.
Summary
Cyberthreats are increasing daily with continuously changing forms, like malware, phishing, crypto-jacking, and ransomware. Due to its ever-changing nature and evolution, it becomes difficult to detect and manage the attacks timely, given the stringent resources, tools, workforce, and knowledge required to mitigate the situation. According to the World Economic Forum's Global Risk Report, the rate of detection (or prosecution) of a cyberattack is as low as 0.05 percent in the U.S. This means that the system remains unpatched, which makes them vulnerable to security breaches, and can be exploited by a malicious attacker (an adversary). There is a huge gap between research needed to develop resource-constrained approaches for detecting and mitigating "organization-specific" security threats. Our researchers have worked to close this gap by developing a decision support tool for detecting and mitigating critical organization-specific cyber threats. This tool would help an organization quantify context-based vulnerability exposure and optimize vulnerability triage and mitigation, keeping in mind the resource containment of the system.
The main components in the system are the dynamic vulnerability priority scoring system (VPSS), deep reinforcement learning (DRL)-based intelligent agent (defender), and DRL-based strategic adversarial agent (adversary). The VPSS considers various organization-specific factors for rating the vulnerability for prioritizing mitigation. This decision depends on the VPSS calculations, scan reports, and human-in-the-loop feedback, among others. The DRL defender assigns the mitigation threat to automated agents or skilled personnel. In addition to assigning threats for mitigation, the DRL adversary performs a red team evaluation of the defender's policies to identify the weaknesses, which are then incorporated into the defender's learning to optimize its security decisions (policies). This organization-specific defending mechanism can help companies to prioritize and mitigate risks and strengthen their security posture.
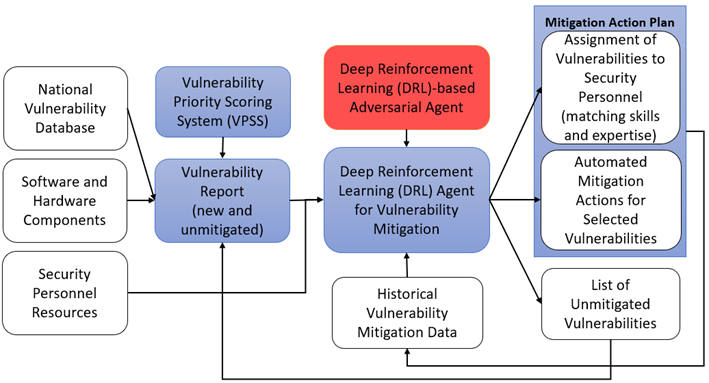
DRL Framework for Defender and Adversarial Evaluation
Desired Partnerships
- License
- Sponsored Research
- Co-Development