Competitive Advantages
- Rank the risk factors with respect to the percent of contribution to the happiness scores
- Identified the significant interactions that influence the happiness score
- This model can be used strategically to increase the degree of happiness
Summary
USF inventors have developed this statistical model with the data available on World Happiness Report 2019 website and the information obtained by a poll. The backward elimination process is used to determine the most significant contributions of both the individual attributable variables and interactions by eliminating the less important risk factors. The statistical estimation process of the data analysis observed that only seven out of the eleven risk factors significantly contribute and twenty-eight interaction terms. The quality of the proposed analytical model is assessed by both the coefficient of determination, R2 and adjusted R2. The performance of the proposed model is compared to the other models (Ridge, LASSO, and ElasticNet) using the error scores RMSE and MAE values. Repeated 10 fold repeated cross-validation for validation testing. To attest the accuracy of the proposed model Min-Max-Accuracy, Correlation Accuracy, SMAPE are calculated.
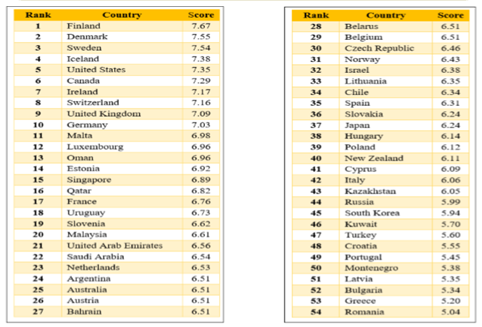
Ranking of The Developed Countries Based On Predicted Happiness Score
Desired Partnerships
- License
- Sponsored Research
- Co-Development