Competitive Advantages
- Adaptable learning
- Flexibility in realistic situations
- Makes real time decisions
Summary
USF inventors have created a trajectory generation approach that learns a broad and stochastic policy from demonstrations. This approach first learns from the human demonstrations of the policies and is able to generate a trajectory with arbitrary length, and treats the change of the constraints naturally. Based on the magnitude of action and the desired future state, the dynamic system decides to keep, reject or execute samples. The approach weighs the action particles drawn from the policy by their respective likelihood of achieving a desired future outcome, and obtains the optimal action using the weighed actions.
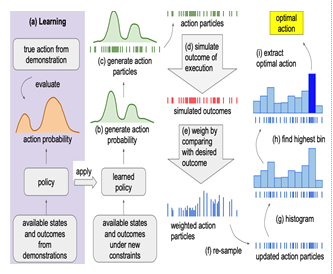
The steps of our approach for trajectory generation
Desired Partnerships
- License
- Sponsored Research
- Co-Development